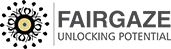
Significance of Algorithmic Bias in Machine Learning
Education News | Apr-20-2024
In the world of technology, and more so, the evolving field of machine learning, everything comes under the rule of algorithms. Over these complex codes, the control that we can exercise is very minimal as they govern everything in our social circles from the friends that we meet to the financial decisions that we make influencing our daily lives in ways that are often beyond our awareness. However, amidst the marvels of automation and artificial intelligence (AI), lies a lurking concern: the problem of algorithmic bias. This delicate process, however, reflects in how the written word is perceived, widening gaps, and in direst cases influencing the very structure of equality and justice.
In this article, we embark on exploring how algorithmic bias can affect machine learning to explain its implications and also create pathways for mitigation.
Understanding Algorithmic Bias:
Algorithmic discrimination is what occurs when machine learning technology becomes systematic and is unfair towards defined groups, where the results highly discriminate in favor of one group over the other. Although algorithms are supposed to process a vast amount of data and make certain objective decisions, they are inherently biased and they may be even mixed with information that is biased or involved in the process of making algorithms. And these biases have several different forms such as race, gender, age, and socio-economic range or geography.
Implications for Society:
Algorithmic bias is an invisible danger that is subtly being integrated into distinctive areas of society, with a devastating effect on communities and the well-being of the affected individuals. In the realm of criminal justice, biased algorithms that are used in risk assessment templates have been widely asserted to lead to minority groups' high risk in combination with legal cycles, thereby, the discrimination is amplified. Equally important in the area of work is the use of biased algorithms employed by recruiters. They might be unintentionally bringing the same demography to the advantage over others from existing workplace disparities.
Furthermore, algorithmic bias is not restricted to areas like healthcare, finance, and education which are crucial for people and turning into obstacles influencing access to significant services and experiences. The healthcare algorithms that are biased in the diagnostics sphere can lead to misdiagnoses or wrong treatments that come to exacerbate the health disparity. In the financial sphere, biased algorithms can be used for credit scoring, which may unfairly prevent customers from a disadvantageous background from getting credit, or impose a higher interest rate on them, reducing their chance to become economically stable faster.
Addressing Algorithmic Bias:
The fight against algorithmic bias involves both a multifaceted approach and attention to data acquisition, algorithm designing, and regulations frameworks. First of all, we have to examine training data and apply cleaning-up procedures to eradicate biases and ensure they exist all across the various demographic groups. Moreover, algorithm engineers must include in their design reliability and openness by creating as transparent and understandable as possible the model’s decision-making process.
Additionally, a diverse and inclusive team working on development can develop more insight into biases and also help design better inclusive algorithms. However, regulatory agencies take the leading role in defining standards and protocols of algorithmic fairness, and they punish developers for violation of guidelines and abortive according to ethical operation and principles.
Conclusion, Algorithmic bias occurs as a great pressure in machine learning subjects which raises fundamental questions like justice, fairness, and equality in human life. Nevertheless, with the increasingly critical decisions that programs have to process, it proves more vital not only to win over and redress the biases that are layered into these systems. Through the nurturing of openness, accountability, and inclusivity during system development, the future can be envisioned where the fairness and equality principles of AI technology become a prevailing reality, and all the goodness of tech advancement can truly be shared by all.
Anand School of Excellence
Related News
-
Augmented Reality (AR) and Virtual Reality (VR) in Education
-
Emerging Trends in Particle Physics
-
Significance of Algorithmic Bias in Machine Learning
-
Significance of Cybersecurity in Critical Infrastructure
-
Importance of Privacy-Preserving Techniques in Data Mining
-
The Evolution of Programming Languages
-
The Physics of Medical Imaging Techniques
-
Environmental Art: Using Creativity to Raise Awareness about Ecological Issues
-
The Future of Blockchain Technology: Beyond Cryptocurrencies
-
The Impact of Mass Media on Public Perception of Social Issues
Most popular news
- The Law Of Equivalent Exchange
- Essay On Issues And Challenges Of Rural Development In India
- Srinivasa Ramanujan And His Inventions
- The Youth Is The Hope Of Our Future!
- Poverty In India: Facts, Causes, Effects And Solutions
- Top 20 Outdoor Games In India
- Festivals Of India: Unity In Diversity
- Role Of Women In Society
- The Impact Of Peer Pressure On Students' Academic Performance
- Books As Companion