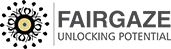
Generalized Feature Embedding for Supervised, Unsupervised & Online Learning Tasks
General News | Jul-04-2023
Generalized feature embedding is a versatile technique that has demonstrated its efficacy in multiple learning tasks, including supervised, unsupervised, and online learning. It involves transforming high-dimensional input data into a lower-dimensional representation that captures relevant and discriminative information.
Supervised learning greatly benefits from generalized feature embedding as it enhances the performance of classification tasks. By mapping input data to a lower-dimensional space, feature embedding algorithms effectively extract pertinent features while reducing noise and irrelevant information. Consequently, the discrimination between different classes improves, leading to a better generalization of unseen data. Applications of supervised learning that leverage feature embedding include image classification, speech recognition, and sentiment analysis.
In unsupervised learning, feature embedding methods enable the discovery of underlying structures and patterns within the data. Techniques like autoencoders and generative adversarial networks (GANs) learn representations that capture the underlying data distribution. These learned embeddings are useful for tasks such as clustering, dimensionality reduction, and anomaly detection. Unsupervised feature embedding empowers researchers to comprehend complex data by revealing compact and meaningful representations.
Furthermore, generalized feature embedding is valuable in online learning scenarios where data arrives in a streaming fashion. Online feature embedding algorithms dynamically update the embeddings as new data arrives, facilitating continuous learning and adaptation. This adaptability is particularly advantageous in applications where the data distribution may change over time, such as online recommendation systems or adaptive personalization.
In conclusion, generalized feature embedding is a powerful technique applicable to various learning tasks. It improves supervised learning by enhancing discrimination and generalization, enables unsupervised learning by uncovering latent structures, and supports online learning by adapting to evolving data. The ability to extract meaningful and compact representations from high-dimensional data makes feature embedding a valuable tool in machine learning applications, contributing to improved performance and comprehension.
Anand School for Excellence
Related News
-
Student Expression Encouraged by Creative Writing Workshops
-
Digital Nomadism: The Internet-Driven Lifestyle Revolution
-
How Gen Z and new generations are shaping social media trends
-
E-Waste: The Environmental Impact of Digital Waste
-
68th National School Games in Jammu: Jharkhand Team Arrives
-
The Importance of Continuous Learning in Today's Job Market
-
Impact of Deforestation and Habitat Fragmentation
-
Essential Soft Skills for Career Advancement
-
How to Cultivate a Growth Mindset at Work
-
Remote Work vs. Office Work: Pros and Cons for Career Growth
Most popular news
- The Law Of Equivalent Exchange
- Essay On Issues And Challenges Of Rural Development In India
- Srinivasa Ramanujan And His Inventions
- The Youth Is The Hope Of Our Future!
- Poverty In India: Facts, Causes, Effects And Solutions
- Top 20 Outdoor Games In India
- Festivals Of India: Unity In Diversity
- Role Of Women In Society
- The Impact Of Peer Pressure On Students' Academic Performance
- Books As Companion