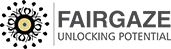
Machine Can Also Learn Without Negative Data
Editorials News | Jan-19-2019
Researcher team has found a method for machine learning that allows AI to make classification without is known as “negative data” a finding which could lead to wider application to a variety of classification tasks.
Further, classifying things critical for our daily life, for example we detect spam mail, fake political news as well as more mundane things such as face and object. When we use AI, such task is totally based on ‘Classification Technology’ in machine learning having the computer learn from the boundary separating positive and negative data. For example ‘positive data would give happy face and negative data will give sad face’. Once the classification boundary is leaned, then the computer can determine whether a certain data is positive or negative, the drawback of this technology is that require both the positive and negative data for the learning process, negative data is not available in many cases. For instance, it is very difficult to find the photo with label, “this photo includes sad face”, since most people smile on the camera.
In the real life program, when the retailer is trying to predict who will make the purchase, it is easy to find the data of the customer who purchased from them but it is very difficult to find the data of the customers who will not purchase from them, since they do not have the access to their competitors data.
Lead author Takashi Ishida from RIKEN AIP, previous classification models couldn’t cope up with the situation where the negative data were not available but we have made possible for the computers to learn only with positive data as long as we have the confidence score for our positive data, from information such as buying intention or the active rate of app users. Using our new method we can let the computer learn a classifier only from positive data equipped with confidence.
Ishida proposed, along with researcher Niu Gang from his group and team leader Masashi Sugiyama, they led the computer learn well by adding the confidence score, which mathematically correspond to the probability where the data belong to the positive class or not .They have succeeded in making the computer learn a classification boundary only from positive data and information on its confidence against the classification problem of machine learning that divide the data positively and negatively.
By: Lakshender S Angras
Content: https://www.sciencedaily.com/releases/2018/11/181126123323.htm
Related News
-
Sustainable Fishing Practices in Goa
-
How Rising CO2 Levels Affect Plant Health and Productivity
-
India's Foreign Policy 2025: Key Relationships and Developments
-
The Dynamics of “The Great Red Sport” of Jupiter
-
Women's Leadership in Times of Crisis
-
"Rekha Gupta: A New Era of Leadership for Delhi!"
-
The Delhi Verdict: Who Will Emerge Victorious?
-
Union Budget 2025: Everything You Need To Know
-
Winners of Ms. & Mrs. India Glam 2025 Announced
-
Period Poverty
Most popular news
- The Law Of Equivalent Exchange
- Essay On Issues And Challenges Of Rural Development In India
- Srinivasa Ramanujan And His Inventions
- The Youth Is The Hope Of Our Future!
- Poverty In India: Facts, Causes, Effects And Solutions
- Top 20 Outdoor Games In India
- Festivals Of India: Unity In Diversity
- Role Of Women In Society
- The Impact Of Peer Pressure On Students' Academic Performance
- Books As Companion