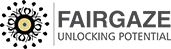
Magnets Can Help The AI Approach The Efficiency Of The Human Brain
Editorials News | May-11-2019
Researchers from Purdue University and brain-inspired computer experts think that part of the answer can be found in magnets. Researchers have developed a process to use magnetic with networks like those of a brain to program and teach devices such as personal robots, automobiles and automatic drones to generalize better about different objects.
"Our stochastic neural networks try to mimic certain activities of the human brain and are computed through a connection of neurons and synapses," said Kaushik Roy, Edward G. Tiedemann Jr., professor of Electrical and Computer Engineering at Purdue. "This allows the brain of the computer not only to store information, but also to generalize well about objects and then make inferences to have a better performance in the distinction between objects."
Roy introduced the technology during the German annual physical science conference earlier this month in Germany. The work also appeared on the borders in neuroscience.
The switching dynamics of a nanoimano is like the electrical dynamics of neurons. The magnetic tunnel junction devices show a switching behavior, which is stochastic in nature
The stochastic change behavior is representative of a sigmoid change behavior of a neuron. Mentioned magnetic tunnel junctions can also be used to store synaptic weights.
The Purdue group proposed a new stochastic training algorithm for synapses using spindle time dependent plasticity (STDP), called Stochastic-STDP, which has been experimentally observed in the hippocampus of the rat. The inherent stochastic behavior of the magnet was used to stochastically change magnetization states based on the proposed algorithm to learn different representations of objects.
The trained synaptic weights, deterministically encoded in the magnetization state of the nanomagnets, are used during the inference. Advantageously, the use of high energy barrier magnets (30-40KT where K is the Boltzmann constant and T is the operating temperature) not only allows compact stochastic primitives, but also allows the use of the same device as an element of Stable memory that meets the retention requirement data. However, the height of the barrier of the nanomagnets used to perform sigmoid-type neural calculations can be reduced to 20 KT for greater energy efficiency.
"The great advantage of the magnet technology we have developed is that it is very energy efficient," said Roy, who runs Purdue's Intelligent Authentic Intelligence Center. "We have created a simpler network that represents neurons and synapses while compressing the amount of memory and energy needed to perform functions similar to those of brain calculations."
By: Preeti Narula
Content: https://www.sciencedaily.com/releases/2019/04/190425104114.htm
Related News
-
"Rekha Gupta: A New Era of Leadership for Delhi!"
-
The Delhi Verdict: Who Will Emerge Victorious?
-
Union Budget 2025: Everything You Need To Know
-
Winners of Ms. & Mrs. India Glam 2025 Announced
-
Period Poverty
-
The World of Jellyfish: Biology, Ecology, and Conservation
-
The Amazing Story of India’s Independence
-
Jaipur LPG Tanker Explosion
-
Maharashtra Elections 2024: BJP-Led Alliance Wins
-
Air and Water Pollution in India 2025: Solutions and Initiatives
Most popular news
- The Law Of Equivalent Exchange
- Essay On Issues And Challenges Of Rural Development In India
- Srinivasa Ramanujan And His Inventions
- The Youth Is The Hope Of Our Future!
- Poverty In India: Facts, Causes, Effects And Solutions
- Top 20 Outdoor Games In India
- Festivals Of India: Unity In Diversity
- Role Of Women In Society
- The Impact Of Peer Pressure On Students' Academic Performance
- Books As Companion